Progressive Deployment of AI-Powered ESG Information Systems
Vogosen recommends progressive deployment of AI-powered ESG information systems. In this article, we discuss the six steps that we take with investors to ensure smooth integration into the investor's investment progress.
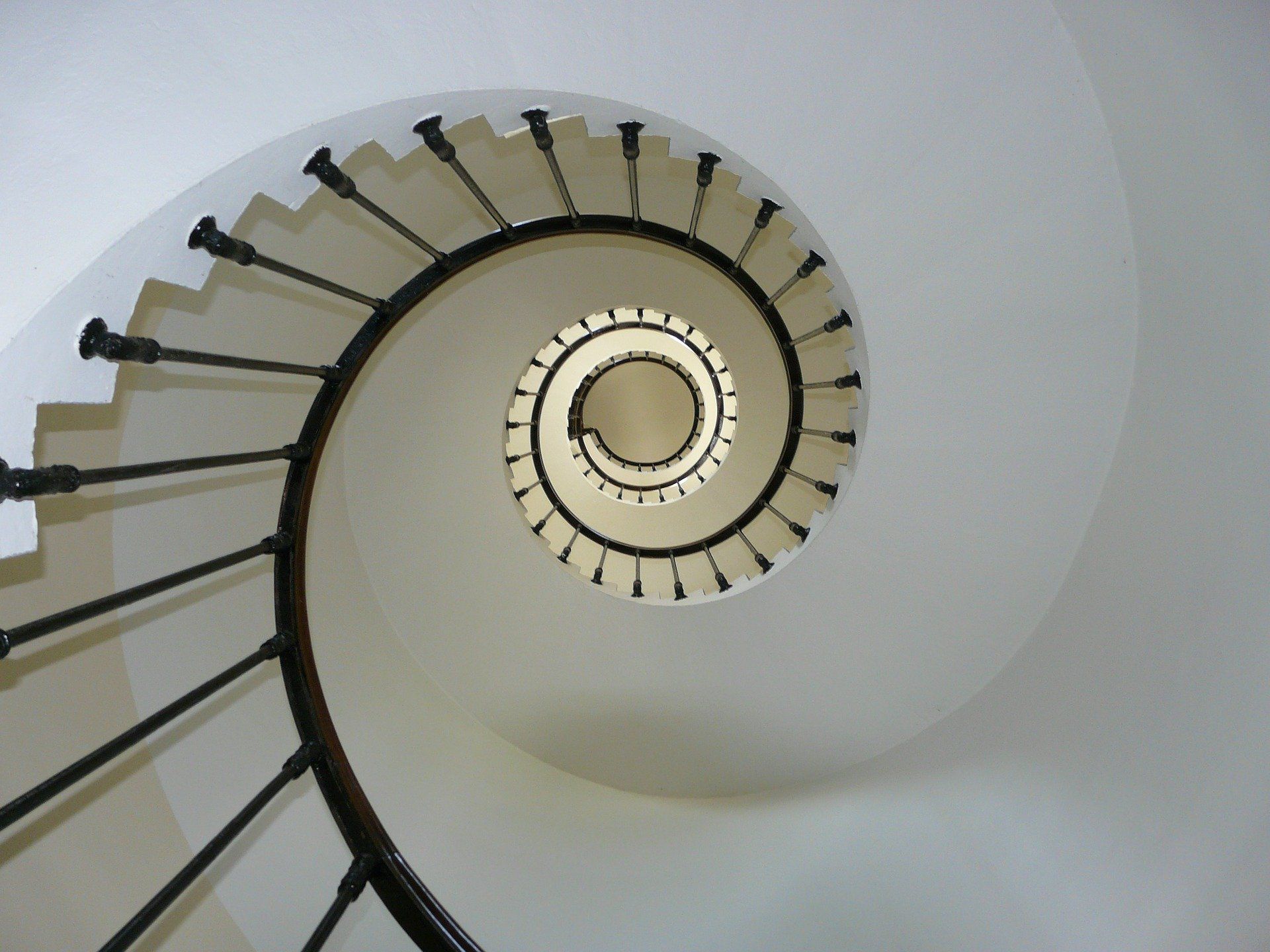
An AI-powered ESG information system, as we have discussed in earlier posts and in our White Paper on AI in ESG, can be configured with a rich set of features and functionalities. However, successful investors are rarely, if ever, naïve in their approach to data: AI-powered ESG components, therefore, must be integrated progressively into the existing infrastructure. Even for investors who lack a strong data infrastructure, we recommend progressive development and deployment of an AI-powered ESG information system.
We recommend progressive deployment of AI-powered ESG information systems not only because these modules have logical dependency on each other but, more importantly, because it is critical to grow relevant organizational capabilities and broaden organizational vision in-house in order to take full advantage of such a new technology.
At Vogosen, we follow six clear steps in an Agile manner so that each step not only prepares for the next step(s) but also foresees future needs.
Six Steps of Progressive Development
First, the investor defines the Objective of the system with us. Initially, the objective may simply be to track material issues of the portfolio more clearly or to monitor a large set of information sources in a timely fashion; over time, new objectives can be added. It is important to define the outputs clearly here: these outputs should be meaningful for your decision-making and/or contain actionable signals.
Second, we identify with the investor Inputs to the system. Some inputs may be readily available, though to interpret them appropriately depends on the objectives of the information system as well as other inputs that must be taken into consideration. Other inputs may not be readily available, and we need to find out how to collect them. Part of these may require subsystems, for example, outputs from other AI modules.
Third, together we iron out the Preprocessing of the inputs so that they are primed for producing the outputs in a clear, unbiased fashion. Indeed, here is also when the investor determines with us whether to adopt machine learning.
Fourth, we work out a solid Data Structure and a set of Algorithms with the investor. We assess how the investor currently manages investment data—that is, data beyond ESG. If the investor has yet to deploy any modern information system and still rely on spreadsheets, we first propose an easy-to-use, cheap-to-implement database system with secure GUI (like spreadsheets but much more powerful) that protects data security, ensures data consistency and integrity, and facilitates data sharing across teams in the organization. Beyond conventional relational (SQL) databases, we use other data storages that are optimized for specific tasks, e.g. Graph DB for semantic queries and ontology.
Fifth, the investor chooses with us the desired User Interface elements, including features of the application such as visualization, that make sense to them.
Sixth, we define a Governance and Maintenance structure with the investor so that the information system is resilient, secure, and efficient. This involves indicators and warning systems that signal issues before they undermine the entire system.